Last active
May 30, 2019 09:03
-
-
Save nitinmlvya/83881bf41a39183ef8ffc95f518f24c5 to your computer and use it in GitHub Desktop.
This file contains bidirectional Unicode text that may be interpreted or compiled differently than what appears below. To review, open the file in an editor that reveals hidden Unicode characters.
Learn more about bidirectional Unicode characters
{ | |
"cells": [ | |
{ | |
"cell_type": "markdown", | |
"metadata": { | |
"slideshow": { | |
"slide_type": "slide" | |
} | |
}, | |
"source": [ | |
"### Feature Extraction:\n", | |
"- Converts a lower dimension features to higher dimension features.\n", | |
"- It creates a new features from the existing features.\n", | |
"- Techniques are one-hot encoding, Bag of words, TFIDF, Word2Vec etc.\n", | |
"\n", | |
"### Feature Selection:\n", | |
"- Removes irrelevent and redundant features.\n", | |
"- Keeps only those features that explains the most of the target variable.\n", | |
"- It is dimension reduction techniques.\n", | |
"- Converts higher dimension features to lower dimensions." | |
] | |
}, | |
{ | |
"cell_type": "markdown", | |
"metadata": { | |
"slideshow": { | |
"slide_type": "slide" | |
} | |
}, | |
"source": [ | |
"### One hot encoding:\n", | |
"It is a process of transforming the categorical variables into numeric that helps ML for better prediction.\n", | |
"Return N-1 categories as features.\n", | |
"### Label Encoding:\n", | |
"It is the process of applying numeric values to categorical variable.\n", | |
"Ex - Male = 0, Female = 1" | |
] | |
}, | |
{ | |
"cell_type": "code", | |
"execution_count": 9, | |
"metadata": { | |
"ExecuteTime": { | |
"end_time": "2019-05-30T08:41:07.134916Z", | |
"start_time": "2019-05-30T08:41:07.114172Z" | |
}, | |
"slideshow": { | |
"slide_type": "subslide" | |
} | |
}, | |
"outputs": [ | |
{ | |
"data": { | |
"text/html": [ | |
"<div>\n", | |
"<style scoped>\n", | |
" .dataframe tbody tr th:only-of-type {\n", | |
" vertical-align: middle;\n", | |
" }\n", | |
"\n", | |
" .dataframe tbody tr th {\n", | |
" vertical-align: top;\n", | |
" }\n", | |
"\n", | |
" .dataframe thead th {\n", | |
" text-align: right;\n", | |
" }\n", | |
"</style>\n", | |
"<table border=\"1\" class=\"dataframe\">\n", | |
" <thead>\n", | |
" <tr style=\"text-align: right;\">\n", | |
" <th></th>\n", | |
" <th>India</th>\n", | |
" <th>UK</th>\n", | |
" <th>US</th>\n", | |
" </tr>\n", | |
" </thead>\n", | |
" <tbody>\n", | |
" <tr>\n", | |
" <th>0</th>\n", | |
" <td>0</td>\n", | |
" <td>1</td>\n", | |
" <td>0</td>\n", | |
" </tr>\n", | |
" <tr>\n", | |
" <th>1</th>\n", | |
" <td>0</td>\n", | |
" <td>1</td>\n", | |
" <td>0</td>\n", | |
" </tr>\n", | |
" <tr>\n", | |
" <th>2</th>\n", | |
" <td>1</td>\n", | |
" <td>0</td>\n", | |
" <td>0</td>\n", | |
" </tr>\n", | |
" <tr>\n", | |
" <th>3</th>\n", | |
" <td>0</td>\n", | |
" <td>0</td>\n", | |
" <td>1</td>\n", | |
" </tr>\n", | |
" <tr>\n", | |
" <th>4</th>\n", | |
" <td>0</td>\n", | |
" <td>1</td>\n", | |
" <td>0</td>\n", | |
" </tr>\n", | |
" </tbody>\n", | |
"</table>\n", | |
"</div>" | |
], | |
"text/plain": [ | |
" India UK US\n", | |
"0 0 1 0\n", | |
"1 0 1 0\n", | |
"2 1 0 0\n", | |
"3 0 0 1\n", | |
"4 0 1 0" | |
] | |
}, | |
"execution_count": 9, | |
"metadata": {}, | |
"output_type": "execute_result" | |
} | |
], | |
"source": [ | |
"# One hot encoding using pandas\n", | |
"one_hot_encoded_custom = pd.get_dummies(df['Country'], columns=df['Country'].unique())\n", | |
"one_hot_encoded_custom.head()" | |
] | |
}, | |
{ | |
"cell_type": "code", | |
"execution_count": 10, | |
"metadata": { | |
"ExecuteTime": { | |
"end_time": "2019-05-30T08:41:08.680326Z", | |
"start_time": "2019-05-30T08:41:07.138284Z" | |
}, | |
"slideshow": { | |
"slide_type": "subslide" | |
} | |
}, | |
"outputs": [ | |
{ | |
"data": { | |
"text/plain": [ | |
"array([1, 1, 0, 0, 1, 1, 0, 0, 1, 0, 0, 0, 1, 1, 1, 1, 1, 1, 1, 0, 1, 0,\n", | |
" 1, 0, 1, 1, 1, 0, 1, 1, 1, 0, 0, 0, 1, 1, 0, 1, 0, 0, 0, 0, 1, 1,\n", | |
" 0, 1, 1, 0, 1, 0, 0, 0, 0, 0, 0, 0, 0, 1, 1, 0, 1, 1, 0, 1, 0, 1,\n", | |
" 1, 0, 0, 0, 1, 0, 0, 0, 1, 1, 1, 0, 0, 0, 1, 1, 1, 1, 0, 0, 1, 0,\n", | |
" 1, 1, 1, 0, 1, 0, 0, 0, 0, 1, 1, 0, 1, 1, 0, 0, 0, 1, 0, 1, 1, 0,\n", | |
" 0, 0, 1, 1, 1, 1, 1, 1, 1, 1, 0, 1, 0, 1, 0, 0, 1, 1, 1, 0, 1, 1,\n", | |
" 1, 0, 0, 1, 0, 1, 0, 1, 1, 0, 1, 1, 0, 0, 0, 0, 1, 1, 0, 1, 1, 0,\n", | |
" 1, 0, 1, 1, 1, 0, 1, 1, 0, 1, 0, 0, 0, 0, 1, 0, 1, 1, 0, 0, 0, 0,\n", | |
" 0, 1, 1, 0, 1, 0, 0, 1, 0, 1, 0, 0, 1, 1, 1, 0, 1, 1, 1, 1, 0, 0,\n", | |
" 1, 1, 1, 1, 0, 0, 0, 0, 0, 0, 0, 0, 0, 1, 0, 1, 1, 0, 1, 1, 0, 1,\n", | |
" 0, 1, 1, 1, 0, 1, 0, 1, 0, 0, 0, 1, 1, 1, 0, 1, 1, 0, 0, 0, 1, 1,\n", | |
" 0, 0, 0, 0, 0, 0, 1, 0, 0, 1, 0, 0, 0, 0, 0, 1, 0, 0, 0, 1, 0, 0,\n", | |
" 1, 0, 1, 1, 0, 1, 0, 0, 1, 1, 0, 1, 1, 1, 0, 0, 0, 1, 1, 0, 1, 0,\n", | |
" 0, 0, 1, 0, 1, 1, 1, 1, 0, 0, 1, 0, 1, 1, 0, 1, 0, 1, 0, 1, 0, 0,\n", | |
" 1, 0, 0, 1, 0, 0, 0, 0, 0, 1, 1, 1, 0, 0, 1, 0, 0, 0, 1, 0, 1, 0,\n", | |
" 1, 0, 1, 1, 1, 0, 1, 1, 0, 1, 0, 1, 0, 0, 1, 0, 1, 0, 1, 1, 0, 1,\n", | |
" 0, 0, 1, 1, 1, 0, 1, 1, 1, 0, 0, 0, 1, 0, 0, 1, 1, 0, 0, 1, 0, 1,\n", | |
" 0, 0, 0, 0, 1, 0, 1, 1, 0, 1, 0, 1, 0, 1, 1, 0, 1, 1, 0, 1, 0, 0,\n", | |
" 1, 0, 1, 0])" | |
] | |
}, | |
"execution_count": 10, | |
"metadata": {}, | |
"output_type": "execute_result" | |
} | |
], | |
"source": [ | |
"# One hot encoding using sklearn\n", | |
"from sklearn.preprocessing import OneHotEncoder, LabelEncoder\n", | |
"label_encoder = LabelEncoder()\n", | |
"le = label_encoder.fit_transform(df['Gender'])\n", | |
"le" | |
] | |
}, | |
{ | |
"cell_type": "code", | |
"execution_count": 11, | |
"metadata": { | |
"ExecuteTime": { | |
"end_time": "2019-05-30T08:41:08.771272Z", | |
"start_time": "2019-05-30T08:41:08.690074Z" | |
}, | |
"scrolled": true, | |
"slideshow": { | |
"slide_type": "skip" | |
} | |
}, | |
"outputs": [ | |
{ | |
"data": { | |
"text/plain": [ | |
"array([[0., 1.],\n", | |
" [0., 1.],\n", | |
" [1., 0.],\n", | |
" [1., 0.],\n", | |
" [0., 1.],\n", | |
" [0., 1.],\n", | |
" [1., 0.],\n", | |
" [1., 0.],\n", | |
" [0., 1.],\n", | |
" [1., 0.],\n", | |
" [1., 0.],\n", | |
" [1., 0.],\n", | |
" [0., 1.],\n", | |
" [0., 1.],\n", | |
" [0., 1.],\n", | |
" [0., 1.],\n", | |
" [0., 1.],\n", | |
" [0., 1.],\n", | |
" [0., 1.],\n", | |
" [1., 0.],\n", | |
" [0., 1.],\n", | |
" [1., 0.],\n", | |
" [0., 1.],\n", | |
" [1., 0.],\n", | |
" [0., 1.],\n", | |
" [0., 1.],\n", | |
" [0., 1.],\n", | |
" [1., 0.],\n", | |
" [0., 1.],\n", | |
" [0., 1.],\n", | |
" [0., 1.],\n", | |
" [1., 0.],\n", | |
" [1., 0.],\n", | |
" [1., 0.],\n", | |
" [0., 1.],\n", | |
" [0., 1.],\n", | |
" [1., 0.],\n", | |
" [0., 1.],\n", | |
" [1., 0.],\n", | |
" [1., 0.],\n", | |
" [1., 0.],\n", | |
" [1., 0.],\n", | |
" [0., 1.],\n", | |
" [0., 1.],\n", | |
" [1., 0.],\n", | |
" [0., 1.],\n", | |
" [0., 1.],\n", | |
" [1., 0.],\n", | |
" [0., 1.],\n", | |
" [1., 0.],\n", | |
" [1., 0.],\n", | |
" [1., 0.],\n", | |
" [1., 0.],\n", | |
" [1., 0.],\n", | |
" [1., 0.],\n", | |
" [1., 0.],\n", | |
" [1., 0.],\n", | |
" [0., 1.],\n", | |
" [0., 1.],\n", | |
" [1., 0.],\n", | |
" [0., 1.],\n", | |
" [0., 1.],\n", | |
" [1., 0.],\n", | |
" [0., 1.],\n", | |
" [1., 0.],\n", | |
" [0., 1.],\n", | |
" [0., 1.],\n", | |
" [1., 0.],\n", | |
" [1., 0.],\n", | |
" [1., 0.],\n", | |
" [0., 1.],\n", | |
" [1., 0.],\n", | |
" [1., 0.],\n", | |
" [1., 0.],\n", | |
" [0., 1.],\n", | |
" [0., 1.],\n", | |
" [0., 1.],\n", | |
" [1., 0.],\n", | |
" [1., 0.],\n", | |
" [1., 0.],\n", | |
" [0., 1.],\n", | |
" [0., 1.],\n", | |
" [0., 1.],\n", | |
" [0., 1.],\n", | |
" [1., 0.],\n", | |
" [1., 0.],\n", | |
" [0., 1.],\n", | |
" [1., 0.],\n", | |
" [0., 1.],\n", | |
" [0., 1.],\n", | |
" [0., 1.],\n", | |
" [1., 0.],\n", | |
" [0., 1.],\n", | |
" [1., 0.],\n", | |
" [1., 0.],\n", | |
" [1., 0.],\n", | |
" [1., 0.],\n", | |
" [0., 1.],\n", | |
" [0., 1.],\n", | |
" [1., 0.],\n", | |
" [0., 1.],\n", | |
" [0., 1.],\n", | |
" [1., 0.],\n", | |
" [1., 0.],\n", | |
" [1., 0.],\n", | |
" [0., 1.],\n", | |
" [1., 0.],\n", | |
" [0., 1.],\n", | |
" [0., 1.],\n", | |
" [1., 0.],\n", | |
" [1., 0.],\n", | |
" [1., 0.],\n", | |
" [0., 1.],\n", | |
" [0., 1.],\n", | |
" [0., 1.],\n", | |
" [0., 1.],\n", | |
" [0., 1.],\n", | |
" [0., 1.],\n", | |
" [0., 1.],\n", | |
" [0., 1.],\n", | |
" [1., 0.],\n", | |
" [0., 1.],\n", | |
" [1., 0.],\n", | |
" [0., 1.],\n", | |
" [1., 0.],\n", | |
" [1., 0.],\n", | |
" [0., 1.],\n", | |
" [0., 1.],\n", | |
" [0., 1.],\n", | |
" [1., 0.],\n", | |
" [0., 1.],\n", | |
" [0., 1.],\n", | |
" [0., 1.],\n", | |
" [1., 0.],\n", | |
" [1., 0.],\n", | |
" [0., 1.],\n", | |
" [1., 0.],\n", | |
" [0., 1.],\n", | |
" [1., 0.],\n", | |
" [0., 1.],\n", | |
" [0., 1.],\n", | |
" [1., 0.],\n", | |
" [0., 1.],\n", | |
" [0., 1.],\n", | |
" [1., 0.],\n", | |
" [1., 0.],\n", | |
" [1., 0.],\n", | |
" [1., 0.],\n", | |
" [0., 1.],\n", | |
" [0., 1.],\n", | |
" [1., 0.],\n", | |
" [0., 1.],\n", | |
" [0., 1.],\n", | |
" [1., 0.],\n", | |
" [0., 1.],\n", | |
" [1., 0.],\n", | |
" [0., 1.],\n", | |
" [0., 1.],\n", | |
" [0., 1.],\n", | |
" [1., 0.],\n", | |
" [0., 1.],\n", | |
" [0., 1.],\n", | |
" [1., 0.],\n", | |
" [0., 1.],\n", | |
" [1., 0.],\n", | |
" [1., 0.],\n", | |
" [1., 0.],\n", | |
" [1., 0.],\n", | |
" [0., 1.],\n", | |
" [1., 0.],\n", | |
" [0., 1.],\n", | |
" [0., 1.],\n", | |
" [1., 0.],\n", | |
" [1., 0.],\n", | |
" [1., 0.],\n", | |
" [1., 0.],\n", | |
" [1., 0.],\n", | |
" [0., 1.],\n", | |
" [0., 1.],\n", | |
" [1., 0.],\n", | |
" [0., 1.],\n", | |
" [1., 0.],\n", | |
" [1., 0.],\n", | |
" [0., 1.],\n", | |
" [1., 0.],\n", | |
" [0., 1.],\n", | |
" [1., 0.],\n", | |
" [1., 0.],\n", | |
" [0., 1.],\n", | |
" [0., 1.],\n", | |
" [0., 1.],\n", | |
" [1., 0.],\n", | |
" [0., 1.],\n", | |
" [0., 1.],\n", | |
" [0., 1.],\n", | |
" [0., 1.],\n", | |
" [1., 0.],\n", | |
" [1., 0.],\n", | |
" [0., 1.],\n", | |
" [0., 1.],\n", | |
" [0., 1.],\n", | |
" [0., 1.],\n", | |
" [1., 0.],\n", | |
" [1., 0.],\n", | |
" [1., 0.],\n", | |
" [1., 0.],\n", | |
" [1., 0.],\n", | |
" [1., 0.],\n", | |
" [1., 0.],\n", | |
" [1., 0.],\n", | |
" [1., 0.],\n", | |
" [0., 1.],\n", | |
" [1., 0.],\n", | |
" [0., 1.],\n", | |
" [0., 1.],\n", | |
" [1., 0.],\n", | |
" [0., 1.],\n", | |
" [0., 1.],\n", | |
" [1., 0.],\n", | |
" [0., 1.],\n", | |
" [1., 0.],\n", | |
" [0., 1.],\n", | |
" [0., 1.],\n", | |
" [0., 1.],\n", | |
" [1., 0.],\n", | |
" [0., 1.],\n", | |
" [1., 0.],\n", | |
" [0., 1.],\n", | |
" [1., 0.],\n", | |
" [1., 0.],\n", | |
" [1., 0.],\n", | |
" [0., 1.],\n", | |
" [0., 1.],\n", | |
" [0., 1.],\n", | |
" [1., 0.],\n", | |
" [0., 1.],\n", | |
" [0., 1.],\n", | |
" [1., 0.],\n", | |
" [1., 0.],\n", | |
" [1., 0.],\n", | |
" [0., 1.],\n", | |
" [0., 1.],\n", | |
" [1., 0.],\n", | |
" [1., 0.],\n", | |
" [1., 0.],\n", | |
" [1., 0.],\n", | |
" [1., 0.],\n", | |
" [1., 0.],\n", | |
" [0., 1.],\n", | |
" [1., 0.],\n", | |
" [1., 0.],\n", | |
" [0., 1.],\n", | |
" [1., 0.],\n", | |
" [1., 0.],\n", | |
" [1., 0.],\n", | |
" [1., 0.],\n", | |
" [1., 0.],\n", | |
" [0., 1.],\n", | |
" [1., 0.],\n", | |
" [1., 0.],\n", | |
" [1., 0.],\n", | |
" [0., 1.],\n", | |
" [1., 0.],\n", | |
" [1., 0.],\n", | |
" [0., 1.],\n", | |
" [1., 0.],\n", | |
" [0., 1.],\n", | |
" [0., 1.],\n", | |
" [1., 0.],\n", | |
" [0., 1.],\n", | |
" [1., 0.],\n", | |
" [1., 0.],\n", | |
" [0., 1.],\n", | |
" [0., 1.],\n", | |
" [1., 0.],\n", | |
" [0., 1.],\n", | |
" [0., 1.],\n", | |
" [0., 1.],\n", | |
" [1., 0.],\n", | |
" [1., 0.],\n", | |
" [1., 0.],\n", | |
" [0., 1.],\n", | |
" [0., 1.],\n", | |
" [1., 0.],\n", | |
" [0., 1.],\n", | |
" [1., 0.],\n", | |
" [1., 0.],\n", | |
" [1., 0.],\n", | |
" [0., 1.],\n", | |
" [1., 0.],\n", | |
" [0., 1.],\n", | |
" [0., 1.],\n", | |
" [0., 1.],\n", | |
" [0., 1.],\n", | |
" [1., 0.],\n", | |
" [1., 0.],\n", | |
" [0., 1.],\n", | |
" [1., 0.],\n", | |
" [0., 1.],\n", | |
" [0., 1.],\n", | |
" [1., 0.],\n", | |
" [0., 1.],\n", | |
" [1., 0.],\n", | |
" [0., 1.],\n", | |
" [1., 0.],\n", | |
" [0., 1.],\n", | |
" [1., 0.],\n", | |
" [1., 0.],\n", | |
" [0., 1.],\n", | |
" [1., 0.],\n", | |
" [1., 0.],\n", | |
" [0., 1.],\n", | |
" [1., 0.],\n", | |
" [1., 0.],\n", | |
" [1., 0.],\n", | |
" [1., 0.],\n", | |
" [1., 0.],\n", | |
" [0., 1.],\n", | |
" [0., 1.],\n", | |
" [0., 1.],\n", | |
" [1., 0.],\n", | |
" [1., 0.],\n", | |
" [0., 1.],\n", | |
" [1., 0.],\n", | |
" [1., 0.],\n", | |
" [1., 0.],\n", | |
" [0., 1.],\n", | |
" [1., 0.],\n", | |
" [0., 1.],\n", | |
" [1., 0.],\n", | |
" [0., 1.],\n", | |
" [1., 0.],\n", | |
" [0., 1.],\n", | |
" [0., 1.],\n", | |
" [0., 1.],\n", | |
" [1., 0.],\n", | |
" [0., 1.],\n", | |
" [0., 1.],\n", | |
" [1., 0.],\n", | |
" [0., 1.],\n", | |
" [1., 0.],\n", | |
" [0., 1.],\n", | |
" [1., 0.],\n", | |
" [1., 0.],\n", | |
" [0., 1.],\n", | |
" [1., 0.],\n", | |
" [0., 1.],\n", | |
" [1., 0.],\n", | |
" [0., 1.],\n", | |
" [0., 1.],\n", | |
" [1., 0.],\n", | |
" [0., 1.],\n", | |
" [1., 0.],\n", | |
" [1., 0.],\n", | |
" [0., 1.],\n", | |
" [0., 1.],\n", | |
" [0., 1.],\n", | |
" [1., 0.],\n", | |
" [0., 1.],\n", | |
" [0., 1.],\n", | |
" [0., 1.],\n", | |
" [1., 0.],\n", | |
" [1., 0.],\n", | |
" [1., 0.],\n", | |
" [0., 1.],\n", | |
" [1., 0.],\n", | |
" [1., 0.],\n", | |
" [0., 1.],\n", | |
" [0., 1.],\n", | |
" [1., 0.],\n", | |
" [1., 0.],\n", | |
" [0., 1.],\n", | |
" [1., 0.],\n", | |
" [0., 1.],\n", | |
" [1., 0.],\n", | |
" [1., 0.],\n", | |
" [1., 0.],\n", | |
" [1., 0.],\n", | |
" [0., 1.],\n", | |
" [1., 0.],\n", | |
" [0., 1.],\n", | |
" [0., 1.],\n", | |
" [1., 0.],\n", | |
" [0., 1.],\n", | |
" [1., 0.],\n", | |
" [0., 1.],\n", | |
" [1., 0.],\n", | |
" [0., 1.],\n", | |
" [0., 1.],\n", | |
" [1., 0.],\n", | |
" [0., 1.],\n", | |
" [0., 1.],\n", | |
" [1., 0.],\n", | |
" [0., 1.],\n", | |
" [1., 0.],\n", | |
" [1., 0.],\n", | |
" [0., 1.],\n", | |
" [1., 0.],\n", | |
" [0., 1.],\n", | |
" [1., 0.]])" | |
] | |
}, | |
"execution_count": 11, | |
"metadata": {}, | |
"output_type": "execute_result" | |
} | |
], | |
"source": [ | |
"ohe = OneHotEncoder(categories='auto')\n", | |
"o_h_e_custom = ohe.fit_transform(le.reshape(-1, 1))\n", | |
"o_h_e_custom.toarray()" | |
] | |
}, | |
{ | |
"cell_type": "markdown", | |
"metadata": { | |
"slideshow": { | |
"slide_type": "slide" | |
} | |
}, | |
"source": [ | |
"### Word Embedding: A method to convert word into numbers.\n", | |
"\n", | |
"**CountVectorizer:** It converts the text document into matrix. It contains frequency of the words in a document. It is also Known as Bag of Words." | |
] | |
}, | |
{ | |
"cell_type": "code", | |
"execution_count": 12, | |
"metadata": { | |
"ExecuteTime": { | |
"end_time": "2019-05-30T08:41:09.327950Z", | |
"start_time": "2019-05-30T08:41:08.780069Z" | |
}, | |
"slideshow": { | |
"slide_type": "subslide" | |
} | |
}, | |
"outputs": [ | |
{ | |
"data": { | |
"text/html": [ | |
"<div>\n", | |
"<style scoped>\n", | |
" .dataframe tbody tr th:only-of-type {\n", | |
" vertical-align: middle;\n", | |
" }\n", | |
"\n", | |
" .dataframe tbody tr th {\n", | |
" vertical-align: top;\n", | |
" }\n", | |
"\n", | |
" .dataframe thead th {\n", | |
" text-align: right;\n", | |
" }\n", | |
"</style>\n", | |
"<table border=\"1\" class=\"dataframe\">\n", | |
" <thead>\n", | |
" <tr style=\"text-align: right;\">\n", | |
" <th></th>\n", | |
" <th>and</th>\n", | |
" <th>document</th>\n", | |
" <th>first</th>\n", | |
" <th>is</th>\n", | |
" <th>one</th>\n", | |
" <th>second</th>\n", | |
" <th>the</th>\n", | |
" <th>third</th>\n", | |
" <th>this</th>\n", | |
" </tr>\n", | |
" </thead>\n", | |
" <tbody>\n", | |
" <tr>\n", | |
" <th>0</th>\n", | |
" <td>0</td>\n", | |
" <td>1</td>\n", | |
" <td>1</td>\n", | |
" <td>1</td>\n", | |
" <td>0</td>\n", | |
" <td>0</td>\n", | |
" <td>1</td>\n", | |
" <td>0</td>\n", | |
" <td>1</td>\n", | |
" </tr>\n", | |
" <tr>\n", | |
" <th>1</th>\n", | |
" <td>0</td>\n", | |
" <td>2</td>\n", | |
" <td>0</td>\n", | |
" <td>1</td>\n", | |
" <td>0</td>\n", | |
" <td>1</td>\n", | |
" <td>1</td>\n", | |
" <td>0</td>\n", | |
" <td>1</td>\n", | |
" </tr>\n", | |
" <tr>\n", | |
" <th>2</th>\n", | |
" <td>1</td>\n", | |
" <td>0</td>\n", | |
" <td>0</td>\n", | |
" <td>1</td>\n", | |
" <td>1</td>\n", | |
" <td>0</td>\n", | |
" <td>1</td>\n", | |
" <td>1</td>\n", | |
" <td>1</td>\n", | |
" </tr>\n", | |
" <tr>\n", | |
" <th>3</th>\n", | |
" <td>0</td>\n", | |
" <td>1</td>\n", | |
" <td>1</td>\n", | |
" <td>1</td>\n", | |
" <td>0</td>\n", | |
" <td>0</td>\n", | |
" <td>1</td>\n", | |
" <td>0</td>\n", | |
" <td>1</td>\n", | |
" </tr>\n", | |
" </tbody>\n", | |
"</table>\n", | |
"</div>" | |
], | |
"text/plain": [ | |
" and document first is one second the third this\n", | |
"0 0 1 1 1 0 0 1 0 1\n", | |
"1 0 2 0 1 0 1 1 0 1\n", | |
"2 1 0 0 1 1 0 1 1 1\n", | |
"3 0 1 1 1 0 0 1 0 1" | |
] | |
}, | |
"execution_count": 12, | |
"metadata": {}, | |
"output_type": "execute_result" | |
} | |
], | |
"source": [ | |
"from sklearn.feature_extraction.text import CountVectorizer\n", | |
"vectorizer = CountVectorizer()\n", | |
"corpus = [\n", | |
" 'This is the first document.',\n", | |
" 'This document is the second document.',\n", | |
" 'And this is the third one.',\n", | |
" 'Is this the first document?',\n", | |
"]\n", | |
"X = vectorizer.fit_transform(corpus)\n", | |
"# print(X.toarray())\n", | |
"# print(vectorizer.get_feature_names())\n", | |
"dfa = pd.DataFrame(X.toarray(), columns=vectorizer.get_feature_names())\n", | |
"dfa" | |
] | |
}, | |
{ | |
"cell_type": "markdown", | |
"metadata": { | |
"slideshow": { | |
"slide_type": "slide" | |
} | |
}, | |
"source": [ | |
"### Tfidf:\n", | |
"- TF-IDF stands for “Term Frequency — Inverse Data Frequency”.\n", | |
"- Term Frequency (tf): gives us the frequency of the word in each document in the corpus.\n", | |
"- Inverse Data Frequency (idf): used to calculate the weight of rare words across all documents in the corpus. The words that occur rarely in the corpus have a high IDF score.\n", | |
"\n", | |
"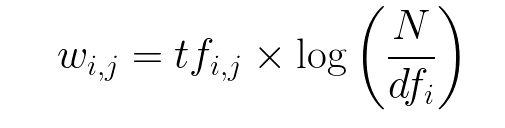", | |
"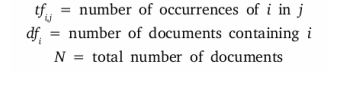" | |
] | |
}, | |
{ | |
"cell_type": "code", | |
"execution_count": 13, | |
"metadata": { | |
"ExecuteTime": { | |
"end_time": "2019-05-30T08:41:09.429141Z", | |
"start_time": "2019-05-30T08:41:09.334439Z" | |
}, | |
"slideshow": { | |
"slide_type": "subslide" | |
} | |
}, | |
"outputs": [ | |
{ | |
"data": { | |
"text/html": [ | |
"<div>\n", | |
"<style scoped>\n", | |
" .dataframe tbody tr th:only-of-type {\n", | |
" vertical-align: middle;\n", | |
" }\n", | |
"\n", | |
" .dataframe tbody tr th {\n", | |
" vertical-align: top;\n", | |
" }\n", | |
"\n", | |
" .dataframe thead th {\n", | |
" text-align: right;\n", | |
" }\n", | |
"</style>\n", | |
"<table border=\"1\" class=\"dataframe\">\n", | |
" <thead>\n", | |
" <tr style=\"text-align: right;\">\n", | |
" <th></th>\n", | |
" <th>and</th>\n", | |
" <th>document</th>\n", | |
" <th>first</th>\n", | |
" <th>is</th>\n", | |
" <th>one</th>\n", | |
" <th>second</th>\n", | |
" <th>the</th>\n", | |
" <th>third</th>\n", | |
" <th>this</th>\n", | |
" </tr>\n", | |
" </thead>\n", | |
" <tbody>\n", | |
" <tr>\n", | |
" <th>0</th>\n", | |
" <td>0.000000</td>\n", | |
" <td>0.469791</td>\n", | |
" <td>0.580286</td>\n", | |
" <td>0.384085</td>\n", | |
" <td>0.000000</td>\n", | |
" <td>0.000000</td>\n", | |
" <td>0.384085</td>\n", | |
" <td>0.000000</td>\n", | |
" <td>0.384085</td>\n", | |
" </tr>\n", | |
" <tr>\n", | |
" <th>1</th>\n", | |
" <td>0.000000</td>\n", | |
" <td>0.687624</td>\n", | |
" <td>0.000000</td>\n", | |
" <td>0.281089</td>\n", | |
" <td>0.000000</td>\n", | |
" <td>0.538648</td>\n", | |
" <td>0.281089</td>\n", | |
" <td>0.000000</td>\n", | |
" <td>0.281089</td>\n", | |
" </tr>\n", | |
" <tr>\n", | |
" <th>2</th>\n", | |
" <td>0.511849</td>\n", | |
" <td>0.000000</td>\n", | |
" <td>0.000000</td>\n", | |
" <td>0.267104</td>\n", | |
" <td>0.511849</td>\n", | |
" <td>0.000000</td>\n", | |
" <td>0.267104</td>\n", | |
" <td>0.511849</td>\n", | |
" <td>0.267104</td>\n", | |
" </tr>\n", | |
" <tr>\n", | |
" <th>3</th>\n", | |
" <td>0.000000</td>\n", | |
" <td>0.469791</td>\n", | |
" <td>0.580286</td>\n", | |
" <td>0.384085</td>\n", | |
" <td>0.000000</td>\n", | |
" <td>0.000000</td>\n", | |
" <td>0.384085</td>\n", | |
" <td>0.000000</td>\n", | |
" <td>0.384085</td>\n", | |
" </tr>\n", | |
" </tbody>\n", | |
"</table>\n", | |
"</div>" | |
], | |
"text/plain": [ | |
" and document first is one second the \\\n", | |
"0 0.000000 0.469791 0.580286 0.384085 0.000000 0.000000 0.384085 \n", | |
"1 0.000000 0.687624 0.000000 0.281089 0.000000 0.538648 0.281089 \n", | |
"2 0.511849 0.000000 0.000000 0.267104 0.511849 0.000000 0.267104 \n", | |
"3 0.000000 0.469791 0.580286 0.384085 0.000000 0.000000 0.384085 \n", | |
"\n", | |
" third this \n", | |
"0 0.000000 0.384085 \n", | |
"1 0.000000 0.281089 \n", | |
"2 0.511849 0.267104 \n", | |
"3 0.000000 0.384085 " | |
] | |
}, | |
"execution_count": 13, | |
"metadata": {}, | |
"output_type": "execute_result" | |
} | |
], | |
"source": [ | |
"from sklearn.feature_extraction.text import TfidfVectorizer\n", | |
"corpus = [\n", | |
" 'This is the first document.',\n", | |
" 'This document is the second document.',\n", | |
" 'And this is the third one.',\n", | |
" 'Is this the first document?',\n", | |
"]\n", | |
"\n", | |
"vectorizer = TfidfVectorizer()\n", | |
"X = vectorizer.fit_transform(corpus)\n", | |
"# print(X.toarray())\n", | |
"# print()\n", | |
"# print(vectorizer.get_feature_names())\n", | |
"dft = pd.DataFrame(X.toarray(), columns=vectorizer.get_feature_names())\n", | |
"dft" | |
] | |
}, | |
{ | |
"cell_type": "markdown", | |
"metadata": { | |
"slideshow": { | |
"slide_type": "slide" | |
} | |
}, | |
"source": [ | |
"### Word2Vec:\n", | |
"- Word2Vec is a shallow, two-layer neural networks which is trained to reconstruct linguistic contexts of words.\n", | |
"- A method that use a vector to represent a word.\n", | |
"- It takes a large corpus of the words and constructs a vector space where each unique word has a vector of hundred of dimensions.\n", | |
"- There are two methods to construct Word2Vec:\n", | |
" - CBOW (Continuous bag of words)\n", | |
" - Skip-gram\n", | |
"- CBOW: It predicts target words (e.g. ‘mat’) from the surrounding context words (‘the cat sits on the’).\n", | |
"- Skip-gram: It predicts surrounding context words from the target words (inverse of CBOW).\n", | |
"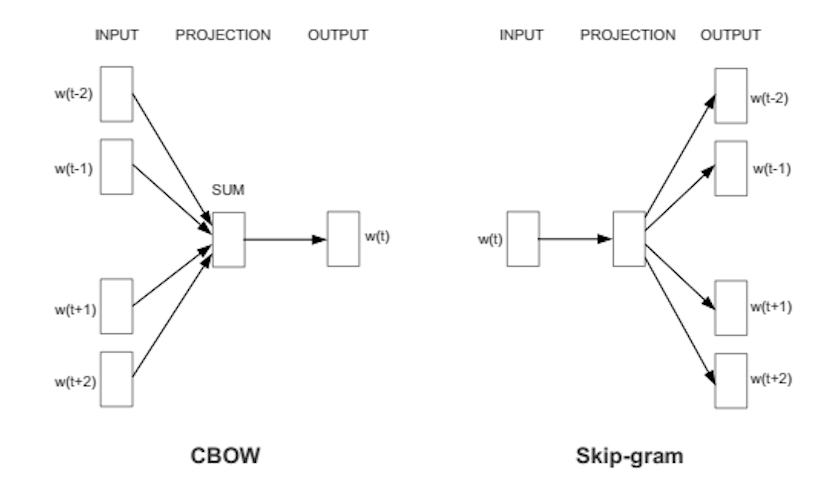" | |
] | |
}, | |
{ | |
"cell_type": "code", | |
"execution_count": 14, | |
"metadata": { | |
"ExecuteTime": { | |
"end_time": "2019-05-30T08:41:10.377608Z", | |
"start_time": "2019-05-30T08:41:09.432080Z" | |
}, | |
"slideshow": { | |
"slide_type": "subslide" | |
} | |
}, | |
"outputs": [ | |
{ | |
"name": "stderr", | |
"output_type": "stream", | |
"text": [ | |
"/usr/local/lib/python3.5/site-packages/ipykernel_launcher.py:22: DeprecationWarning: Call to deprecated `most_similar` (Method will be removed in 4.0.0, use self.wv.most_similar() instead).\n" | |
] | |
}, | |
{ | |
"data": { | |
"text/plain": [ | |
"[('batsman', 0.16431209444999695),\n", | |
" ('took', 0.15877550840377808),\n", | |
" ('by', 0.1548101305961609),\n", | |
" ('owner', 0.15064845979213715),\n", | |
" ('7', 0.14024271070957184),\n", | |
" ('2011', 0.13814853131771088),\n", | |
" ('soundpronunciation', 0.1339716911315918),\n", | |
" ('Year', 0.12925764918327332),\n", | |
" ('resigning', 0.1263372004032135),\n", | |
" ('records', 0.12402260303497314)]" | |
] | |
}, | |
"execution_count": 14, | |
"metadata": {}, | |
"output_type": "execute_result" | |
} | |
], | |
"source": [ | |
"import gensim\n", | |
"from nltk.tokenize import sent_tokenize\n", | |
"from nltk.tokenize import word_tokenize\n", | |
"\n", | |
"text = '''\n", | |
"Mahendra Singh Dhoni (About this soundpronunciation (help·info) born 7 July 1981), commonly known as MS Dhoni, is an Indian international cricketer who captained the Indian national team in limited-overs formats from 2007 to 2016 and in Test cricket from 2008 to 2014. Under his captaincy, India won the 2007 ICC World Twenty20, the 2010 and 2016 Asia Cups, the 2011 ICC Cricket World Cup and the 2013 ICC Champions Trophy. A right-handed middle-order batsman and wicket-keeper.[2][3][4][5] He is also regarded as one of the best wicket-keepers in world cricket.[6][7]\n", | |
"\n", | |
"He made his One Day International (ODI) debut in December 2004 against Bangladesh, and played his first Test a year later against Sri Lanka. Dhoni has been the recipient of many awards, including the ICC ODI Player of the Year award in 2008 and 2009 (the first player to win the award twice), the Rajiv Gandhi Khel Ratna award in 2007, the Padma Shri, India's fourth highest civilian honour, in 2009 and the Padma Bhushan, India's third highest civilian honour, in 2018.[8] He was named as the captain of the ICC World Test XI in 2009, 2010 and 2013. He has also been selected a record 8 times in ICC World ODI XI teams, 5 times as captain. The Indian Territorial Army conferred the honorary rank of Lieutenant Colonel[9] to Dhoni on 1 November 2011. He is the second Indian cricketer after Kapil Dev to receive this honour.\n", | |
"\n", | |
"Dhoni also holds numerous captaincy records such as the most wins by an Indian captain in Tests, ODIs and T20Is, and most back-to-back wins by an Indian captain in ODIs. He took over the ODI captaincy from Rahul Dravid in 2007 and led the team to its first-ever bilateral ODI series wins in Sri Lanka and New Zealand. In June 2013, when India defeated England in the final of the Champions Trophy in England, Dhoni became the first captain to win all three ICC limited-overs trophies (World Cup, Champions Trophy and the World Twenty20). After taking up the Test captaincy in 2008, he led the team to series wins in New Zealand and the West Indies, and the Border-Gavaskar Trophy in 2008, 2010 and 2013. In 2009, Dhoni also led the Indian team to number one position for the first time in the ICC Test rankings.\n", | |
"\n", | |
"In 2013, under his captaincy, India became the first team in more than 40 years to whitewash Australia in a Test series. In the Indian Premier League, he captained the Chennai Super Kings to victory at the 2010, 2011 and 2018 seasons, along with wins in the 2010 and 2014 editions of Champions League Twenty20. In 2011, Time magazine included Dhoni in its annual Time 100 list as one of the \"Most Influential People in the World.\"[10] Dhoni holds the post of Vice-President of India Cements Ltd., after resigning from Air India. India Cements is the owner of the IPL team Chennai Super Kings, and Dhoni has been its captain since the first IPL season.[11][12] He announced his retirement from Tests on 30 December 2014.[13]\n", | |
"\n", | |
"In 2012, SportsPro rated Dhoni as the sixteenth most marketable at\n", | |
"'''\n", | |
"\n", | |
"sentences = sent_tokenize(text)\n", | |
"tokens = [word_tokenize(sent) for sent in sentences]\n", | |
"\n", | |
"model = gensim.models.Word2Vec(tokens, min_count=1,size=300,workers=4)\n", | |
"model.wv.vocab # Vocabulary\n", | |
"model.most_similar('cricket')\n" | |
] | |
}, | |
{ | |
"cell_type": "markdown", | |
"metadata": { | |
"slideshow": { | |
"slide_type": "slide" | |
} | |
}, | |
"source": [ | |
"### Model Evalution:\n", | |
"##### Confusion Matrix:\n", | |
"- Confusion matrix is used to summarize, describe or evaluate the performance of a Binary classification task or model.\n", | |
" - Positive (P) : Actual is positive (for example: is an apple).\n", | |
" - Negative (N): Actual is not positive (for example: is not an apple).\n", | |
" - True Positive (TP): Actual is positive, and is predicted to be positive.\n", | |
" - False Negative (FN): Actual is positive, but is predicted negative.\n", | |
" - True Negative (TN): Actual is negative, and is predicted to be negative.\n", | |
" - False Positive (FP): Actual is negative, but is predicted positive.\n", | |
"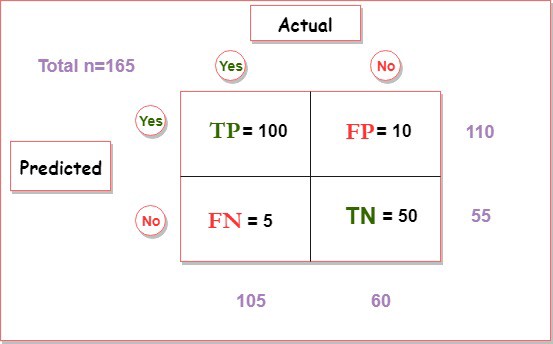" | |
] | |
}, | |
{ | |
"cell_type": "code", | |
"execution_count": 15, | |
"metadata": { | |
"ExecuteTime": { | |
"end_time": "2019-05-30T08:41:10.791245Z", | |
"start_time": "2019-05-30T08:41:10.390282Z" | |
}, | |
"slideshow": { | |
"slide_type": "subslide" | |
} | |
}, | |
"outputs": [ | |
{ | |
"data": { | |
"text/plain": [ | |
"(<Figure size 432x288 with 1 Axes>,\n", | |
" <matplotlib.axes._subplots.AxesSubplot at 0x7fbffe809b00>)" | |
] | |
}, | |
"execution_count": 15, | |
"metadata": {}, | |
"output_type": "execute_result" | |
}, | |
{ | |
"data": { | |
"image/png": "iVBORw0KGgoAAAANSUhEUgAAARMAAAEUCAYAAAAIrNIAAAAABHNCSVQICAgIfAhkiAAAAAlwSFlzAAALEgAACxIB0t1+/AAAADl0RVh0U29mdHdhcmUAbWF0cGxvdGxpYiB2ZXJzaW9uIDMuMC4zLCBodHRwOi8vbWF0cGxvdGxpYi5vcmcvnQurowAAEY9JREFUeJzt3X2UHXV9x/H3BwIaaDRGEKE08qCAiDZUKIiAqQdQK4Kt+ECDiIhQi6J9ULFY0bbUo017etRajMUWGw1RsEXxSKtiqQYRIg8BK8GWB1sFa9RKwIo8fPvHneiCyeYu/O7O3uT9OmfPzp2Ze+ezZ89+duZ3586kqpCkh2uLvgNI2jRYJpKasEwkNWGZSGrCMpHUhGUiqQnLRFITlomkJiwTSU3M6jvAw5FZsytbz+k7hqZg3yfP7zuCpuDWW29hzZo1GWbd8S6TrefwiD1f0ncMTcGKr7yv7wiagmcesN/Q63qYI6kJy0RSE5aJpCYsE0lNWCaSmrBMJDVhmUhqwjKR1IRlIqkJy0RSE5aJpCYsE0lNWCaSmrBMJDVhmUhqwjKR1IRlIqkJy0RSE5aJpCYsE0lNWCaSmrBMJDVhmUhqwjKR1IRlIqkJy0RSE5aJpCYsE0lNWCaSmrBMJDVhmUhqwjKR1IRlIqkJy0RSE5aJpCYsE0lNWCaSmrBMJDVhmUhqwjKR1IRlIqkJy0RSE5aJpCYsE0lNWCaSmrBMJDVhmUhqwjKR1IRlMoPsvMNcLl5yGlddcAZfPf8MTj12Yd+RtBGnnHQi83d6HE9fsE/fUXo348okycIkB/Wdow/33nc/p//lJ/iVF53Fs45fzCkvPZS9dnt837E0iZe/4gQuvOjivmPMCDOuTICFwGZZJrevuYNrbvhvAO780d3ccPPt7LT93J5TaTIHH3Io8+bN6zvGjDBtZZLk+CSrklyb5B+SvCDJV5JcneRzSXZIsgvw28DvJrkmySHTlW+mmb/jPBbsuTNXXn9L31Gkocyajo0keQrwVuCgqlqTZB5QwIFVVUlOAt5UVb+f5GzgzqpavIHXOhk4GYCtfmE64k+7bWdvzbLFJ/HGxRew9q4f9x1HGsq0lAnwbODjVbUGoKq+n+SpwPIkOwJbAzcP80JVtQRYArDFNo+rEeXtzaxZW7Bs8atZ/pmVXHjJtX3HkYbW55jJe4H3VdVTgVOAR/aYZcY4+8xFrL75dt6z9JK+o0hTMl1lcgnw4iSPBegOcx4NfKtb/ooJ664F5kxTrhnloAW7sejIA3jW/ntw+Xmnc/l5p/Ocg/fuO5Ymcfxxx7LwkGdw4+rV7L7Lzvz9h87pO1JvpuUwp6q+luQs4NIk9wFXA28HPp7kBwzKZtdu9U8B5yc5GnhdVX1xOjLOBJddcxOz931t3zE0BR9euqzvCDPGdI2ZUFXnAuc+aPaF61nvRuBp0xJKUjMz8TwTSWPIMpHUhGUiqQnLRFITlomkJiwTSU1YJpKasEwkNWGZSGrCMpHUhGUiqQnLRFITlomkJiwTSU1YJpKasEwkNWGZSGrCMpHUhGUiqQnLRFITlomkJiwTSU1YJpKasEwkNWGZSGrCMpHUhGUiqYkN3ms4yVqg1j3svlc3XVX1qBFnkzRGNlgmVTVnOoNIGm9DHeYkOTjJK7vp7ZLsOtpYksbNRsskyZnAm4G3dLO2BpaOMpSk8TPMnslvAEcBdwFU1bcBD4EkPcAwZfKTqiq6wdgk2442kqRxNEyZfCzJB4C5SV4NfA744GhjSRo3G3w3Z52qWpzkcOAOYA/gbVX12ZEnkzRWNlomneuA2QwOda4bXRxJ42qYd3NOAq4AfhM4Brg8yYmjDiZpvAyzZ/JGYN+q+h5AkscClwEfGmUwSeNlmAHY7wFrJzxe282TpJ+a7LM5v9dN/gfwlSQXMhgzORpYNQ3ZJI2RyQ5z1p2Y9p/d1zoXji6OpHE12Qf93jGdQSSNt40OwCbZHngT8BTgkevmV9WzR5hL0pgZZgD2I8ANwK7AO4BbgCtHmEnSGBqmTB5bVecA91TVpVV1IuBeiaQHGOY8k3u677cleT7wbWDe6CJJGkfDlMmfJnk08PvAe4FHAb870lSSxs4wH/S7qJv8IfBro40jaVxNdtLae/nZBaV/TlWdNpJEU7Dvk+ez4ivv6zuGpuAx+7+27wiagrtXf3PodSfbM1n58KNI2lxMdtLaudMZRNJ48yZckpqwTCQ1YZlIamKYK63tkeTzSa7vHj8tyVtHH03SOBlmz+SDDG7AdQ9AVa0CXjbKUJLGzzBlsk1VXfGgefeOIoyk8TVMmaxJsjs/uwnXMcBtI00laewM89mcU4ElwF5JvgXcDBw30lSSxs4wn825CTisuy3oFlW1dmPPkbT5GeZKa2970GMAquqPR5RJ0hga5jDnrgnTjwSOBL4+mjiSxtUwhzl/MfFxksXAP48skaSx9FDOgN0G2Ll1EEnjbZgxk+v42XVNtgS2BxwvkfQAw4yZHDlh+l7gO1XlSWuSHmDSMkmyJfDPVbXXNOWRNKYmHTOpqvuA1UnmT1MeSWNqmMOcxwBfS3IFE94mrqqjRpZK0tgZpkz+aOQpJI29Ycrk16vqzRNnJHkXcOloIkkaR8OcZ3L4euY9r3UQSeNtsvvmvAb4HWC3JKsmLJoDrBh1MEnjZbLDnI8CnwHeCZw+Yf7aqvr+SFNJGjuT3TfnhwxuCXrs9MWRNK68Or2kJiwTSU1YJpKasEwkNWGZSGrCMpHUhGUiqQnLRFITlomkJiwTSU1YJpKasEwkNWGZSGrCMpHUhGUiqQnLRFITlomkJiwTSU1YJpKasEwkNWGZSGrCMpHUhGUiqQnLRFITlskMcspJJzJ/p8fx9AX79B1FQ9p5h7lcvOQ0rrrgDL56/hmceuzCviP1ZtrKJMnbk/zBdG1vHL38FSdw4UUX9x1DU3Dvffdz+l9+gl950Vk86/jFnPLSQ9lrt8f3HasX7pnMIAcfcijz5s3rO4am4PY1d3DNDf8NwJ0/upsbbr6dnbaf23Oqfoy0TJKckeTGJF8C9uzmLUhyeZJVSf4xyWO6+ft3865J8udJrh9lNqm1+TvOY8GeO3Pl9bf0HaUXIyuTJE8HXgYsAH4d2L9b9GHgzVX1NOA64Mxu/t8Bp1TVAuC+SV735CQrk6z87prvjiq+NCXbzt6aZYtP4o2LL2DtXT/uO04vRrlncgjwj1X1o6q6A/gksC0wt6ou7dY5Fzg0yVxgTlV9uZv/0Q29aFUtqar9qmq/7bfbfoTxpeHMmrUFyxa/muWfWcmFl1zbd5zeOGYiPUxnn7mI1TffznuWXtJ3lF6Nskz+DXhhktlJ5gAvAO4CfpDkkG6dlwOXVtX/AmuTHNDNf9kIc81Yxx93LAsPeQY3rl7N7rvszN9/6Jy+I2kjDlqwG4uOPIBn7b8Hl593OpefdzrPOXjvvmP1YtaoXriqrkqyHLgW+B/gym7RK4Czk2wD3AS8spv/KuCDSe4HLgV+OKpsM9WHly7rO4Km6LJrbmL2vq/tO8aMMLIyAaiqs4Cz1rPowPXM+1o3KEuS04GVo8wmqa2RlskUPT/JWxhkuhU4od84kqZixpRJVS0HlvedQ9JD47s5kpqwTCQ1YZlIasIykdSEZSKpCctEUhOWiaQmLBNJTVgmkpqwTCQ1YZlIasIykdSEZSKpCctEUhOWiaQmLBNJTVgmkpqwTCQ1YZlIasIykdSEZSKpCctEUhOWiaQmLBNJTVgmkpqwTCQ1YZlIasIykdSEZSKpCctEUhOWiaQmLBNJTVgmkpqwTCQ1YZlIasIykdSEZSKpCctEUhOWiaQmLBNJTVgmkpqwTCQ1YZlIasIykdSEZSKpCctEUhOpqr4zPGRJvgvc2neOEdgOWNN3CE3Jpvo7e0JVbT/MimNdJpuqJCurar++c2h4/s48zJHUiGUiqQnLZGZa0ncATdlm/ztzzERSE+6ZSGrCMpHUhGUiqQnLRBqBJOk7w3SzTGaYJFtOmJ7TZxZNXZJdAaqqNrdC8d2cGaQrksOAu4GnAfcDZ1fVvb0G06SSpCuPJwGfBJZW1VkTl/WbcHrM6juAHiDAo4A3AY8Bjqiqe5NsUVX39xtNG9IVydHAy4ErgJck2aqq3r5uD2VzKBTLZAbpiuMK4CfAZcBeSW6rqv/rOZomkWQu8Fbg94AVwFOB9ye5u6reuTkUCThmMqMk2aGqbgWeDXwGOBJ4Ybds7ySP7zOfNug+Bp8Yvqnbg7weWAq8KslpvSabRpbJDJHktcDSJH8OvKSqlgErgYOSnAcsAzaL/3AzWTrd9E5JHlFVa4HLgQuSzK6q+4D/YvAP4fAke/cYedp4mDMDJDkBOBZYBLwbOCLJjlX17iQHAQuBP6mq7/SXUjAYHwFI8lzgTOAb3cD5HzIo+6uSnAOcxmAMZRGbyT9ty6RnSfYD1jI4pFnEYAD2NOBdSWZV1Z8xGD9Rj5JsDxwO/BODwfH3AK8CvsPgUPSjwHOBG4GtgOcBc4D9gDt6iDztLJMeJXkNcATwRga/i8OA46pqTZJvAwcm2a6qNsUreI2N7rDmCAZjWbOAq4HPV9UXu3fa3p3kCcBRVfWR7jn7A38FvLKqvtlX9ulkmfQkyVHAa4AXVNWtSXZksFeyR5IjGZxjcqJF0r/u0OYj3QD4gcBjgaOTXFFVf9et9j1g4gD5/wAvrKrbpzdtfyyT/uwEnNcVyVZVdVuSTwOvA+YDp1okM0eS5wBHAVsCc4GPAX/c/RO4oVv2hnXrd+/KbVY8A7YnSZ4HvB54fVWt7uYdyeDCxMs9t2TmSPI44BPAyVX170lOBXboFj8RuAm4vKou6ivjTOCeSX9WAAcBJyRZweC/3euBYy2SGeceBn8r23WPlwB/DewKLAfO2ZzOdN2QzeItq5moqu4A3s/gVh2/AzwfeFVV/UevwfRzquoHDA5rFibZp6ruAS4A7gK+tK5ANuciAQ9zZoQkWwNU1U/6zqL1S7Iz8NvArwJXAscwGNf6XK/BZhDLRBpSd0mIZwD7AF+tqkt7jjSjWCaSmnDMRFITlomkJiwTSU1YJpKasEwkNWGZaGhJ7uy+75Tk/I2s+4Yk20zx9Rcm+blT0jc0/0HrnJDkfVPc3i1Jttv4mhqGZbKZm3hrjWFV1ber6piNrPYGYEplovFmmWyikuyS5IYkH0ny9STnr9tT6P4jvyvJVcCLk+ye5OIkX03yxSR7devtmuTLSa5L8qcPeu3ru+ktkyxOcn2SVUle1133dCfgC0m+0K13RPdaVyX5eJJf6OY/t8t5FfCbQ/xcv9q9ztVJLkuy54TFv5TkX5N8I8mZE55zXJIrklyT5AMPpUA1hKryaxP8AnZhcBnBZ3aPPwT8QTd9C/CmCet+HnhSN30AcEk3/Ung+G76VODOCa99fTf9GuB8YFb3eN6EbWzXTW8H/Buwbff4zcDbgEcyuFbqkxjc5uNjwEXr+VkWrpvP4Jov67Z1GHBBN30CcBuDa43MZnBR5/2AJwOfArbq1nv/hJ/ppxn9evhffmp40/ZfVbWim17K4HKQi7vHywG6PYSDgI/nZzege0T3/ZnAi7rpfwDetZ5tHMaEG4VV1ffXs86BwN7Aim4bWwNfBvYCbq6qb3RZlgInb+RnejRwbgY3vCoGl0hc57NV9b3utT4BHAzcCzwduLLb9mwGFy5SY5bJpu3Bn5WY+Piu7vsWwP9W1YIhX+OhCIM/9GMfMDPZ0DYn8yfAF6rqN5LsAvzrhGXr+3kDnFtVb3kI29IUOGayaZuf5Bnd9G8BX3rwCjW4FMLNSV4MP72Vwy93i1cAL+umF21gG58FTkkyq3v+vG7+WgYXVIbBbSCemeSJ3TrbJtmDwRXKdkmye7feA8pmAx4NfKubPuFByw5PMi/JbAYXeV7B4BDumO4CR3TLnzDEdjRFlsmmbTVwapKvM7ii+t9sYL1FDG4YdS3wNeDobv7ru+dfB/ziBp77t8A3gVXd83+rm78EuDjJF6rquwz+8JclWUV3iFNVP2ZwWPPpbgB2mMOPdwPvTHI1P79nfQWD64ysYjCWsrKq/p3B3fb+pdv2Z4Edh9iOpshPDW+iukOAi6pqn56jaDPhnomkJtwzkdSEeyaSmrBMJDVhmUhqwjKR1IRlIqkJy0RSE/8P/F+/SC8AYbQAAAAASUVORK5CYII=\n", | |
"text/plain": [ | |
"<Figure size 432x288 with 1 Axes>" | |
] | |
}, | |
"metadata": { | |
"needs_background": "light" | |
}, | |
"output_type": "display_data" | |
} | |
], | |
"source": [ | |
"import numpy as np\n", | |
"from sklearn.metrics import confusion_matrix\n", | |
"from mlxtend.plotting import plot_confusion_matrix\n", | |
"\n", | |
"y_true = np.array(['cat', 'dog', 'cat', 'dog', 'cat', 'dog'])\n", | |
"y_pred = np.array(['cat', 'dog', 'dog', 'cat', 'cat', 'dog'])\n", | |
"classes = np.unique(y_true)\n", | |
"conf_matrix = confusion_matrix(y_true, y_pred)\n", | |
"plot_confusion_matrix(conf_mat=conf_matrix, class_names=classes)\n" | |
] | |
}, | |
{ | |
"cell_type": "markdown", | |
"metadata": { | |
"slideshow": { | |
"slide_type": "slide" | |
} | |
}, | |
"source": [ | |
"### Accuracy\n", | |
" :It is a metric for evaluating classification models. It is the number of correct predictions made divided by the total number of predictions made, multiplied by 100 to turn it into a percentage.\n", | |
"(TP+TN)/Total = (100+50)/165 = 0.91 = 91% \n", | |
"\n", | |
"### Classification Report:\n", | |
"\n", | |
"**Precision:** True positive / Number of predicted positive.\n", | |
"TP / (TP +FP) = 100 / (100+10) = 0.90 = 90% \n", | |
"\n", | |
"**Recall:** True positive / Number of actual positive\n", | |
"TP / (TP + FN) = 100 / (100 + 5) = 0.95 = 95%\n", | |
"\n", | |
"**F1 Score:** It is harmonic mean of precision and recall.\n", | |
"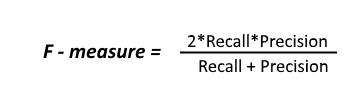", | |
"0.924 = 92.4%\n", | |
"\n", | |
"**Support:** The number of occurrences of each label in y_true." | |
] | |
}, | |
{ | |
"cell_type": "code", | |
"execution_count": 16, | |
"metadata": { | |
"ExecuteTime": { | |
"end_time": "2019-05-30T08:41:10.804168Z", | |
"start_time": "2019-05-30T08:41:10.794182Z" | |
}, | |
"slideshow": { | |
"slide_type": "subslide" | |
} | |
}, | |
"outputs": [ | |
{ | |
"name": "stdout", | |
"output_type": "stream", | |
"text": [ | |
" precision recall f1-score support\n", | |
"\n", | |
" cat 0.67 0.67 0.67 3\n", | |
" dog 0.67 0.67 0.67 3\n", | |
"\n", | |
" micro avg 0.67 0.67 0.67 6\n", | |
" macro avg 0.67 0.67 0.67 6\n", | |
"weighted avg 0.67 0.67 0.67 6\n", | |
"\n" | |
] | |
} | |
], | |
"source": [ | |
"from sklearn.metrics import classification_report\n", | |
"print(classification_report(y_true, y_pred))\n", | |
"\n", | |
"# macro average (averaging the unweighted mean per label)\n", | |
"# weighted average (averaging the support-weighted mean per label)" | |
] | |
} | |
], | |
"metadata": { | |
"celltoolbar": "Slideshow", | |
"kernelspec": { | |
"display_name": "Python 3", | |
"language": "python", | |
"name": "python3" | |
}, | |
"language_info": { | |
"codemirror_mode": { | |
"name": "ipython", | |
"version": 3 | |
}, | |
"file_extension": ".py", | |
"mimetype": "text/x-python", | |
"name": "python", | |
"nbconvert_exporter": "python", | |
"pygments_lexer": "ipython3", | |
"version": "3.5.6" | |
}, | |
"varInspector": { | |
"cols": { | |
"lenName": 16, | |
"lenType": 16, | |
"lenVar": 40 | |
}, | |
"kernels_config": { | |
"python": { | |
"delete_cmd_postfix": "", | |
"delete_cmd_prefix": "del ", | |
"library": "var_list.py", | |
"varRefreshCmd": "print(var_dic_list())" | |
}, | |
"r": { | |
"delete_cmd_postfix": ") ", | |
"delete_cmd_prefix": "rm(", | |
"library": "var_list.r", | |
"varRefreshCmd": "cat(var_dic_list()) " | |
} | |
}, | |
"types_to_exclude": [ | |
"module", | |
"function", | |
"builtin_function_or_method", | |
"instance", | |
"_Feature" | |
], | |
"window_display": false | |
} | |
}, | |
"nbformat": 4, | |
"nbformat_minor": 2 | |
} |
Sign up for free
to join this conversation on GitHub.
Already have an account?
Sign in to comment